

Previously in we used a spatial prior on the valid tissue and resection locations to help estimate these regions.
E TALOS PRINCIPLE IMAGE REGISTRATION
The general joint registration and segmentation framework follows the method described in. The addition of the indicator map into the registration estimation allows the incorporation of different models for observing the data under different correspondence assumptions. In this paper, we present a joint registration and segmentation method in which an indicator function separating the valid and missing correspondence regions is estimated along with the transformation parameters. We are proposing a probabilistically-grounded approach to the simultaneous registration and resection estimation problem. Their method alternated between registering the images using a demons algorithm with an anisotropic diffusion smoother and segmenting the resection by evolving a level set in the region with high intensity errors. An algorithm to accommodate resection and retraction was presented in for alignment of preoperative to intraoperative brain images. Biomechanical models of brain tumor growth and estimation of tissue loss and replacement have been used to improve the registration accuracy around the tumor region.
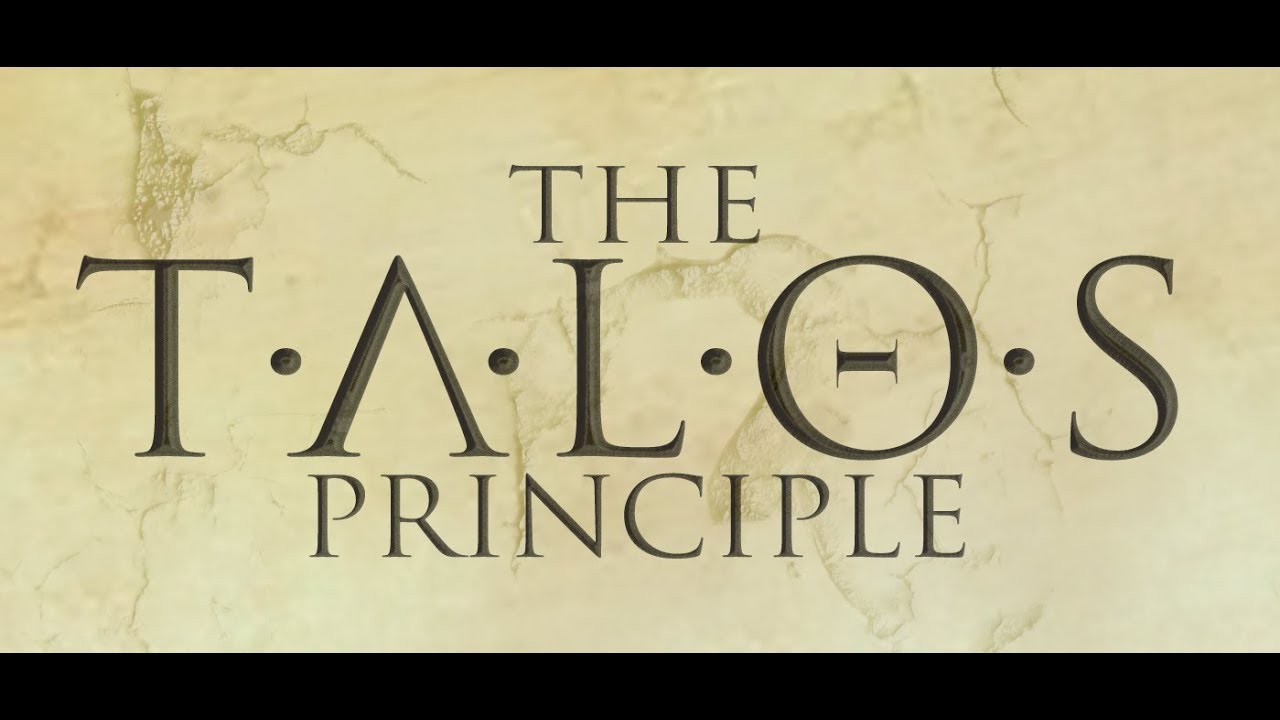
The correspondence problem in the alignment of dynamic contrast-enhanced breast MRI was handled by “de-enhancing” the contrast images before registration in.
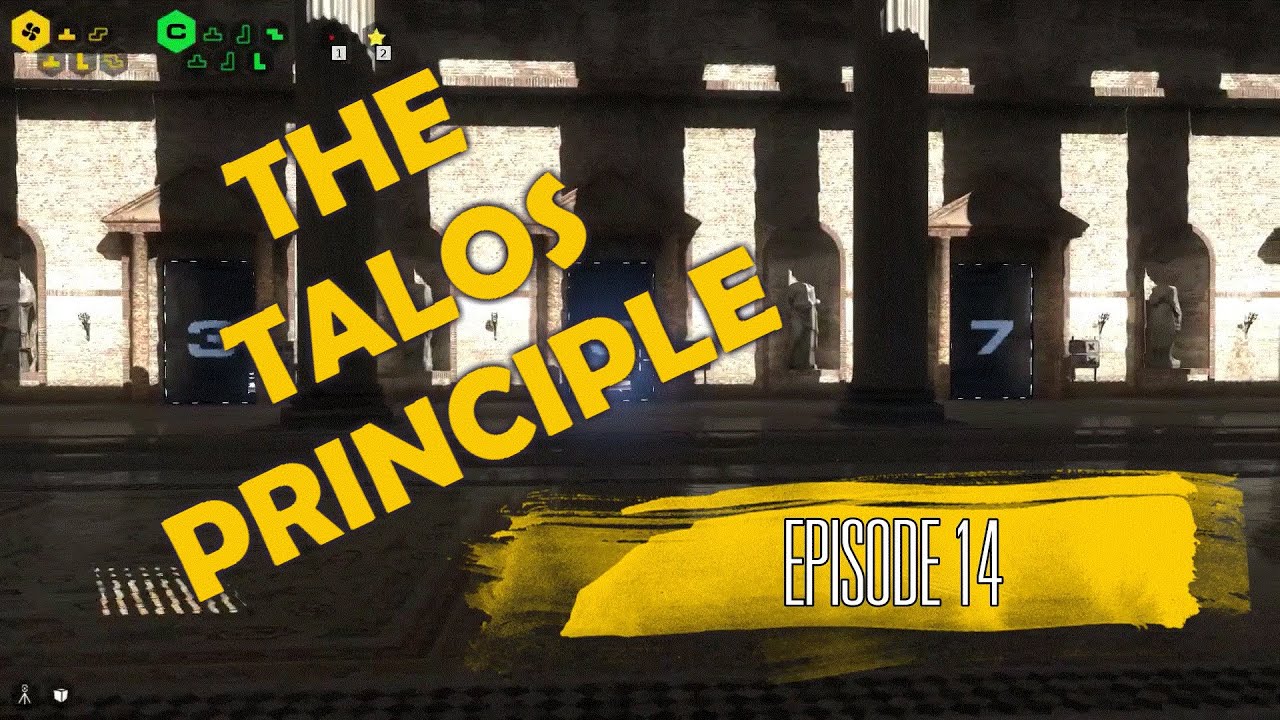
Some application-specific methods have also been proposed to better register images with substantial changes. For our application, it is known that resection voxels will appear dark in T1-weighted MRI due to cerebrospinal fluid (CSF) taking place of the missing tissue, and we will thus incorporate this prior information. Each voxel is assumed to have an equal chance of being labeled as missing or valid data. A method for general registration problems was presented in which dealt with partial data by using an EM-style method to simultaneously estimate the registration parameters and the missing data. While many traditional non-rigid registration algorithms are available, few handle the problem of missing correspondences. This registration problem is challenging due to the missing correspondences caused by resected tissue coupled with the possibility for highly nonlinear deformations in the postresection brain. To better understand epilepsy and the changes that occur in the brain after resection, preoperative and postresection images first need to be aligned. In localization-related epilepsy, brain resection is often considered the next line of treatment if anticonvulsive medication is unable to control seizures.
